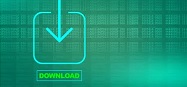
Quantization architecture: Leaky ReLU activated layers, for example, have proven difficult to Note that activations other than ReLU may not work for the encoder and decoder layers in the That their capacity is a good fit for the MNIST dataset. Now for the encoder and the decoder for the VQ-VAE. Thanks to this videoįor helping me understand this technique. Included in the computation graph and th gradients obtaind for quantized During backpropagation, (quantized - x) won't be
CODEBOOK PYTHON CODE
This line of code does the straight-through estimation part: quantized = x + argmin ( distances, axis = 1 ) return encoding_indices embeddings ** 2, axis = 0 ) - 2 * similarity ) # Derive the indices for minimum distances. reduce_sum ( flattened_inputs ** 2, axis = 1, keepdims = True ) + tf. stop_gradient ( quantized - x ) return quantized def get_code_indices ( self, flattened_inputs ): # Calculate L2-normalized distance between the inputs and the codes. beta * commitment_loss + codebook_loss ) # Straight-through estimator. stop_gradient ( quantized ) - x ) ** 2 ) codebook_loss = tf. Check # the original paper to get a handle on the formulation of the loss function. You can learn more # about adding losses to different layers here: #. reshape ( quantized, input_shape ) # Calculate vector quantization loss and add that to the layer. embeddings, transpose_b = True ) # Reshape the quantized values back to the original input shape quantized = tf. get_code_indices ( flattened ) encodings = tf.
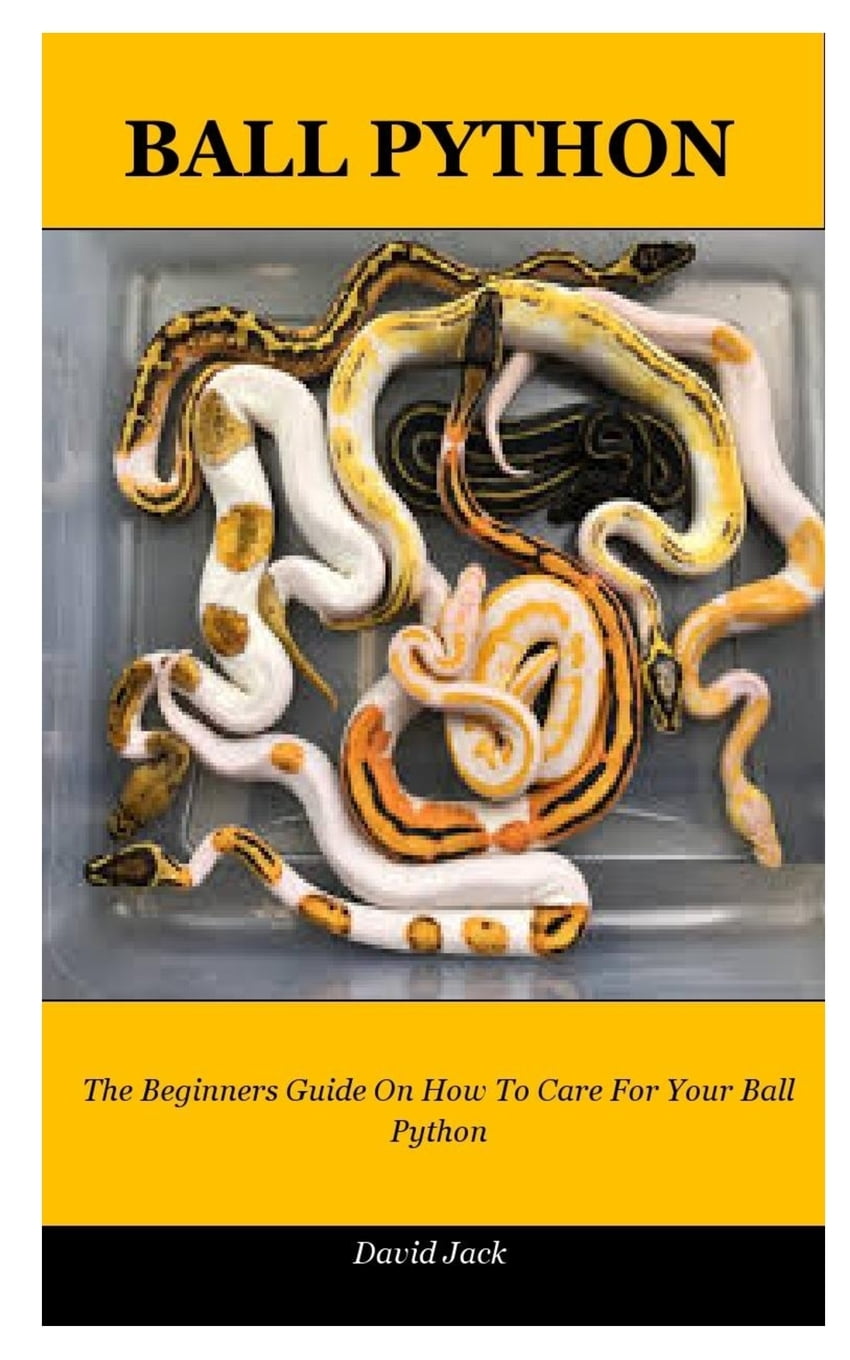
num_embeddings ), dtype = "float32" ), trainable = True, name = "embeddings_vqvae", ) def call ( self, x ): # Calculate the input shape of the inputs and # then flatten the inputs keeping `embedding_dim` intact. Variable ( initial_value = w_init ( shape = ( self.
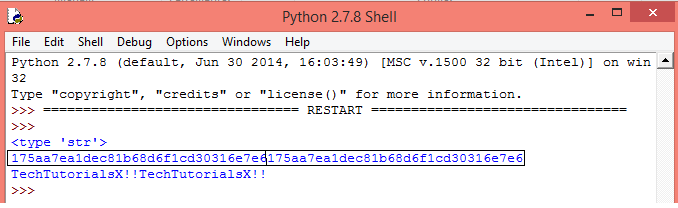
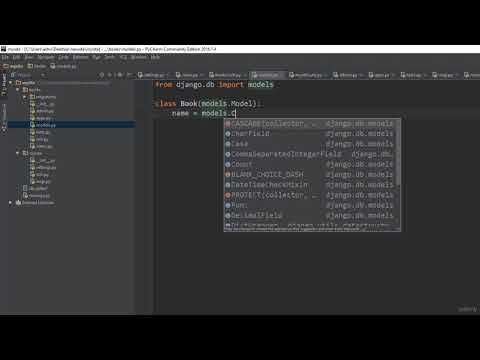
beta = beta # Initialize the embeddings which we will quantize. num_embeddings = num_embeddings # The `beta` parameter is best kept between as per the paper. Layer ): def _init_ ( self, num_embeddings, embedding_dim, beta = 0.25, ** kwargs ): super (). As the encoder and decoder share the same channel space, the decoder gradients areĬlass VectorQuantizer ( layers. In between the decoder and the encoder, so that the decoder gradients are directly propagated Since the quantization process is not differentiable, we apply a Mapped as one and the remaining codes are mapped as zeros. This way, the code yielding the minimum distance to the corresponding encoder output is We take theĬode that yields the minimum distance, and we apply one-hot encoding to achieve quantization. We measure the L2-normalizedĭistance between the flattened encoder outputs and code words of this codebook. The rationale behind this is to treat the total number of filters as the size forĪn embedding table is then initialized to learn a codebook. So, the shape would become (batch_size * height * width, The vector quantizer will first flatten this output, only keeping the Consider an output from the encoder, with shape (batch_size, height, width, Import numpy as np import matplotlib.pyplot as plt from tensorflow import keras from tensorflow.keras import layers import tensorflow_probability as tfp import tensorflow as tfįirst, we implement a custom layer for the vector quantizer, which is the layer in between TensorFlow Probability, which can be installed using the command below. To run this example, you will need TensorFlow 2.5 or higher, as well as This example uses implementation details from the VQ-VAEs are one of the main recipes behind DALL-EĪnd the idea of a codebook is used in VQ-GANs. If you need a refresher on VAEs, you can refer to These discrete code words are then fed to the decoder, which is trainedįor an overview of VQ-VAEs, please refer to the original paper and The codebook is developed byĭiscretizing the distance between continuous embeddings and the encoded It does soīy maintaining a discrete codebook. Operate on a discrete latent space, making the optimization problem simpler. It is generally harder to learn such a continuousĭistribution via gradient descent. In standard VAEs, the latent space is continuous and is sampledįrom a Gaussian distribution.

In this example, we develop a Vector Quantized Variational Autoencoder (VQ-VAE).īy van der Oord et al. Vector-Quantized Variational Autoencodersĭescription: Training a VQ-VAE for image reconstruction and codebook sampling for generation.
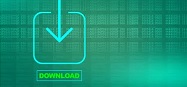